Wenbo Ouyang PhD Defense 08/01/23 3pm
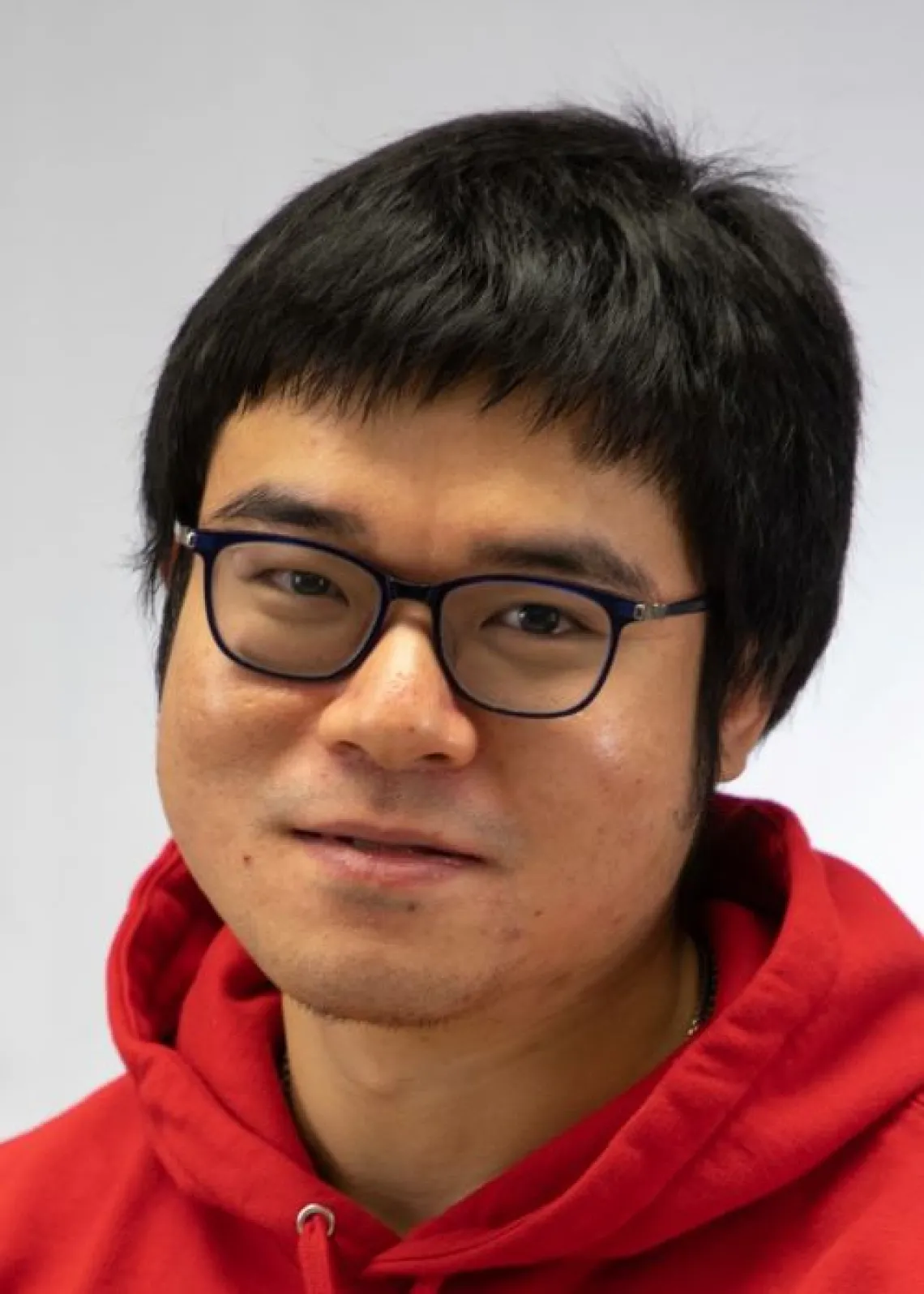
Title: Dynamic Supervised Principal Component Analysis for Classification
Abstract: Contemporary research places great importance on high-dimensional classification, with dynamic classification problems being of particular interest. Such problems involve situations where the distributions of both classes are not static, but change with time or other index variables. This project proposes a new framework in the context of linear discriminant analysis (LDA) for learning classification decision rules that can adapt to changes with respect to the index variable. Furthermore, many existing works on high-dimensional classification problems make the sparsity assumption about the original feature space, which may not hold in practice. Our framework relaxes this assumption by learning the hidden sparse structure of the data through data rotation. In this work, we propose a new dimension reduction method in the context of dynamic problems. The new method employs a kernel smoothing procedure to determine the suitable direction for dimension reduction. Numerical simulations and real data examples are illustrated to demonstrate the performance of the new approach in terms of both classification accuracy and computational efficiency.
Zoom link: https://arizona.zoom.us/j/3475452238